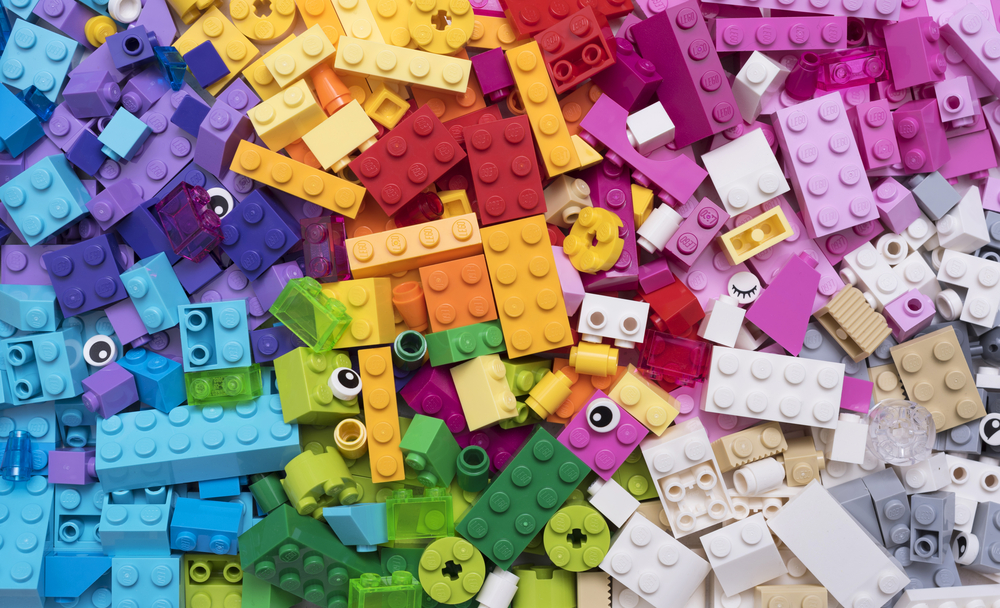
Plenty of parents have had the misfortune of stepping on their kids’ forgotten Lego bricks while barefoot. But Dutch coder
Jacques Mattheij had another mishap involving the colorful plastic building blocks—namely, unintentionally winning more than two tons of them in a late night eBay bidding spree.
To make the best of his situation, Mattheij created a machine learning process to sort the thousands upon thousands of bulk Lego pieces by shape and color. Machine learning, a term computer scientist Arthur Samuel coined in 1959, allows computers to recognize patterns and “learn” to analyze data without being explicitly programmed to do so.
Mattheij set up a system of conveyor belts and bins, through which the bricks traveled “
past a camera paired with a computer running a neural net-based image classifier,” according to Mental Floss. Neural nets, or artificial neural networks, typically comprise several computers linked together. They are designed to mimic the neurons of the human brain in order to teach the computers how to make decisions like a person.
Once Mattheij’s algorithm trained the computer to recognize the Lego bricks by certain parameters, the conveyor belt system could drop the pieces into separate bins. The
computer program also constantly updates as it obtains more data about the Lego pieces, including variations for broken, faded, and imitation Lego blocks.
Experiments in machine learning and the arts
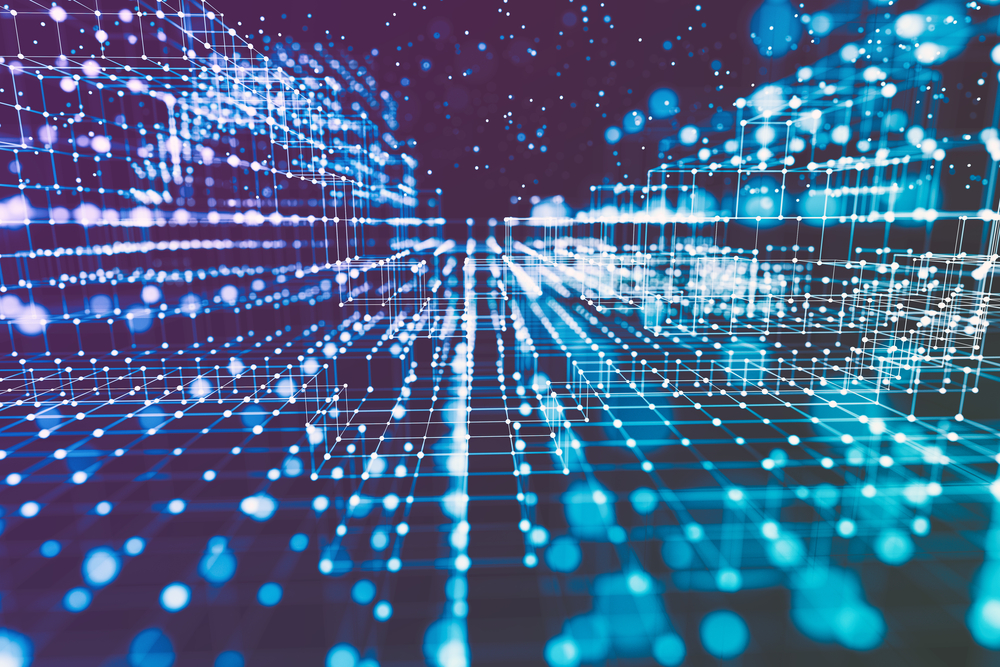
The results of machine learning may sound like science fiction, but they are fast becoming part of our everyday lives. The algorithms that make up machine learning processes help Amazon predict your next purchases and Netflix recommend movies and TV shows. The search engines we use, like Google, use natural language processing—another form of machine learning—to determine the intent behind our queries.
Machine learning is even teaching computers how to watch movies and extrapolate data from them. The Geena Davis Institute on Gender in Media, along with help from Google, recently used machine learning to track
gender bias in the top live-action films of 2014, 2015, and 2016.
Previously, researchers had to watch films one by one and log the results—a labor-intensive task. With machine learning, however, the computers could watch films, categorize the faces on screen, and analyze whether speaking characters were men or women.
In addition to the gender bias study, the film industry has also taken advantage of machine learning with the help of IBM’s Watson. Watson, which competed and won on
Jeopardy! in 2011, analyzed 100 horror films to create a unique movie trailer.
Google’s machine learning
experiments are even infiltrating the arts realm. Users can doodle in Autodraw and have Google complete it—for instance, making a crudely drawn cat actually look like a cat—or try Google’s QuickDraw app to help the machine learn how to recognize said drawings. Google also has apps that let users play a piano duet with a machine, create song lyrics from photos, and utilize an “infinite drum machine” to create a cacophony of everyday sounds sorted by machine learning.
Machine learning in the business world
But machine learning processes do more than serve pop culture. They can even impact the average workplace.
The
Harvard Business Review found that corporate investment in artificial intelligence (AI) is predicted to triple this year and become a $100 billion market by 2025. Businesses are using this technology in everything from customer service and client retention to human resources and supply chain logistics.
In 2017, EagleView acquired OmniEarth, a developer of machine learning technologies and decision-making tools for the water resource management, energy infrastructure, and insurance markets. These technologies have the capability to identify property and land features seen in geospatial imagery.
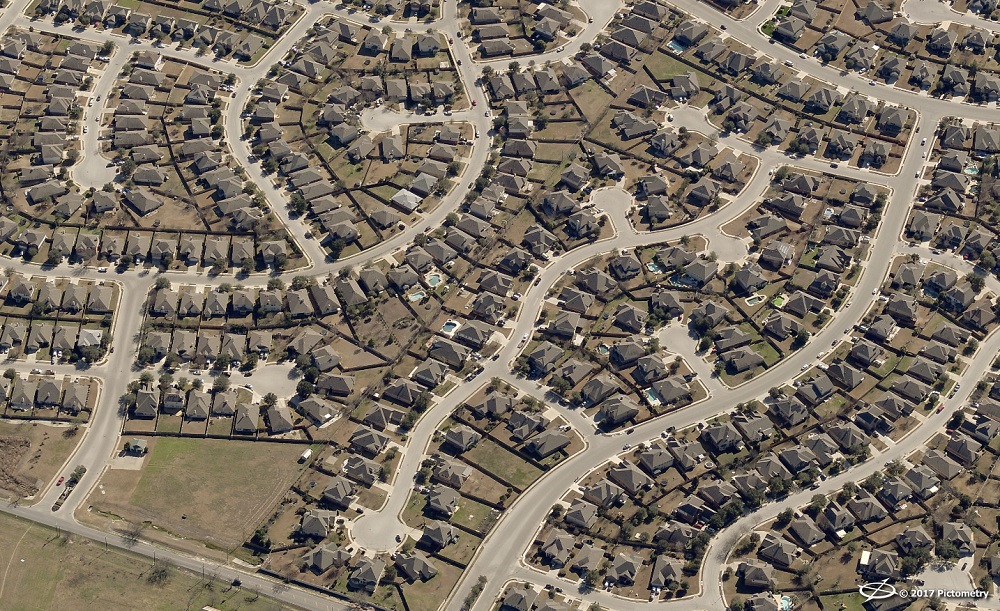
Machine learning technologies can help EagleView identify roof condition, tree overhang, and other features in its Pictometry imagery.
EagleView’s
Pictometry® imagery database totals hundreds of millions of images and more than 60 petabytes (60,000 terabytes) of data. Using these assets alongside these new machine learning technologies, EagleView can more quickly pull data from that imagery. Such features include roof condition and material, tree overhang, vegetation obstructions, and the presence of pools.
Automated processes derived from machine learning are fast making tedious sorting, categorizing, and analyzing a thing of the past. By embracing these advances, we can more quickly obtain data to help with crucial decision-making, whether it revolves around what to do with two tons of Lego bricks or calculating risks and condition for millions of properties.