Change Detection and Disaster Response with Aerial Imagery Using Generative AI
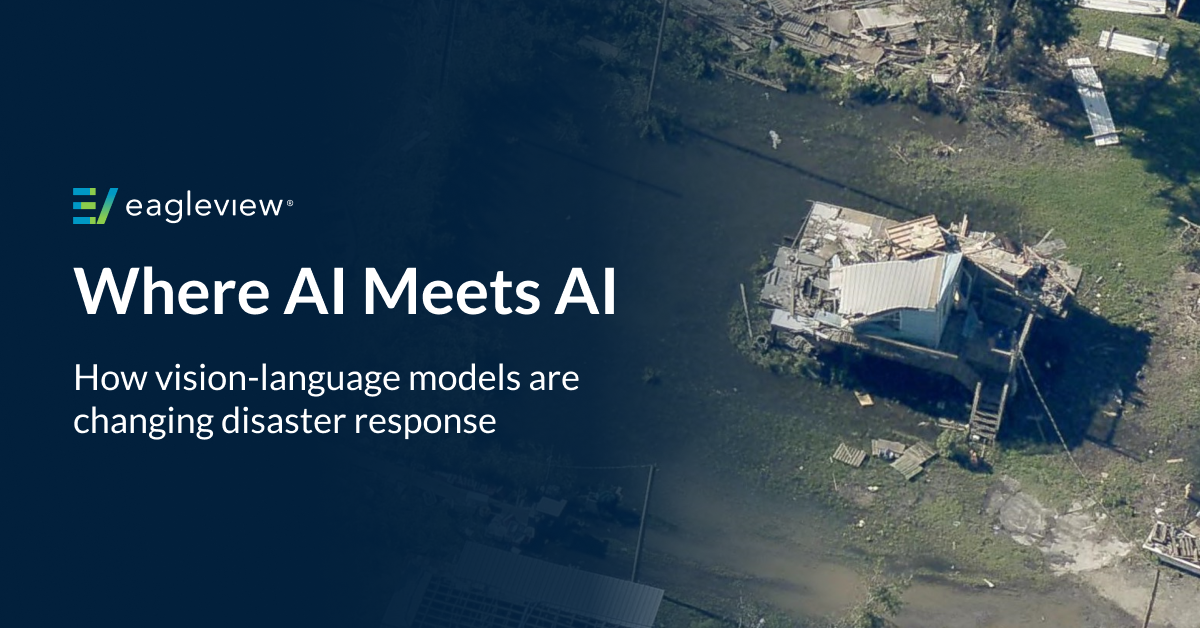
Aerial imagery has become an essential tool in monitoring environmental changes, tracking urban development, and responding to natural disasters. With advancements in generative AI, Vision-Language Models (VLMs) are now playing a crucial role in automating the process of change detection and disaster assessment at EagleView. These models, which integrate visual understanding with natural language processing, offer a more flexible and efficient approach to analyze aerial imagery.
In this blog, we explore how VLMs can be leveraged for change detection and disaster response using aerial imagery.
The Need for Change Detection and Disaster Response
Disasters such as floods, earthquakes, hurricanes, and wildfires can cause significant damage, requiring immediate action. Traditional methods of damage assessment and change detection involve manual inspection of satellite and aerial images, which is time-consuming and prone to errors.
Automated change detection using AI can:
- Identify affected areas rapidly
- Help prioritize rescue and relief efforts
- Reduce manual workload for disaster response teams
- Enhance accuracy in damage assessment
VLMs, which can process both images and textual descriptions, are particularly well-suited for this task as they allow users to query images using natural language, making analysis more accessible and interpretable.
EagleView's Competitive Edge with High-Resolution, Multi-Angle Aerial Imagery
One of EagleView’s key advantages in change detection and disaster response lies in its high-resolution, multi-angle aerial imagery. Unlike traditional satellite images, which often have lower resolution and limited perspectives, EagleView captures up to 1-inch (2.5cm) ground sampling distance resolution with exceptional detail.
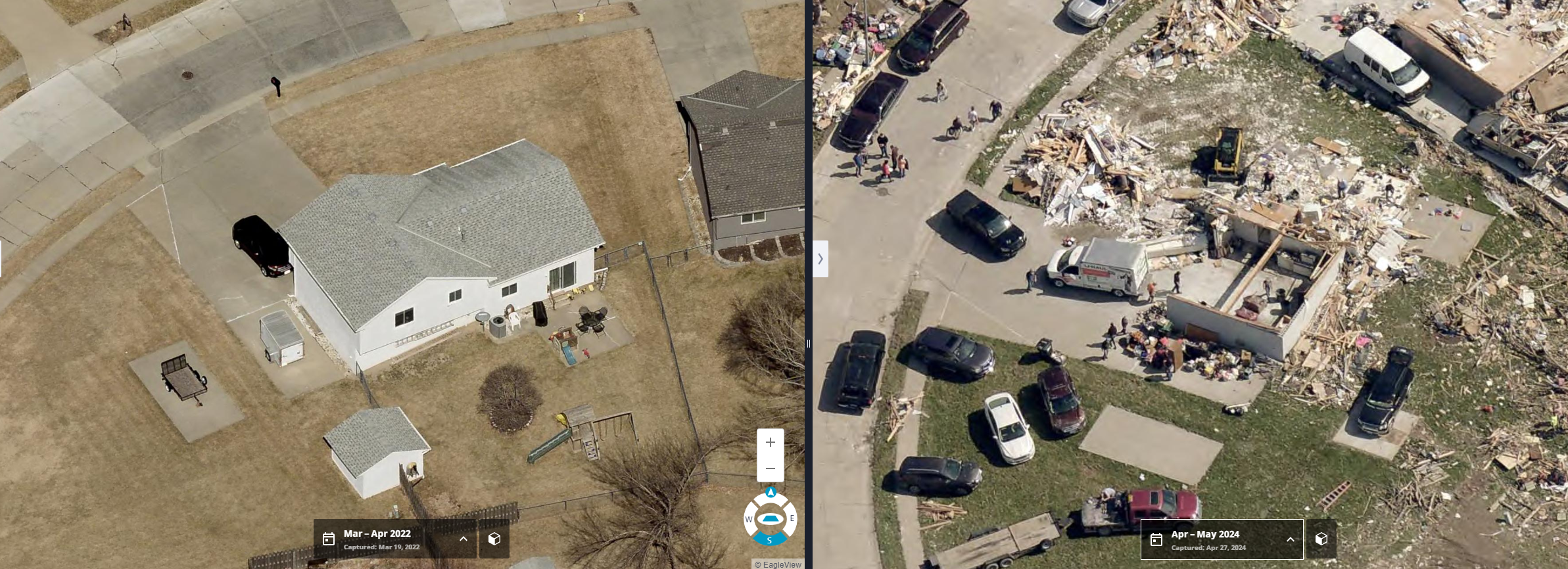
What sets EagleView apart is its ability to capture not only top-down (orthogonal) views but also oblique (angled) imagery. This multi-angle coverage provides a comprehensive 3D-like perspective, enabling the detection of changes not just on roofs but also on walls, windows, and building facades. This level of detail is particularly valuable for post-disaster assessments, where damage to vertical structures is just as critical as rooftop changes.
How Vision-Language Models Work for Aerial Imagery
VLMs are trained on large-scale image-text datasets. These models are designed to:
- Understand and generate captions for images.
- Compare images based on textual descriptions.
- Detect changes between two images using natural language queries.
Here is an example showing the use of EagleView’s VLM Model to identify changes in the imagery:
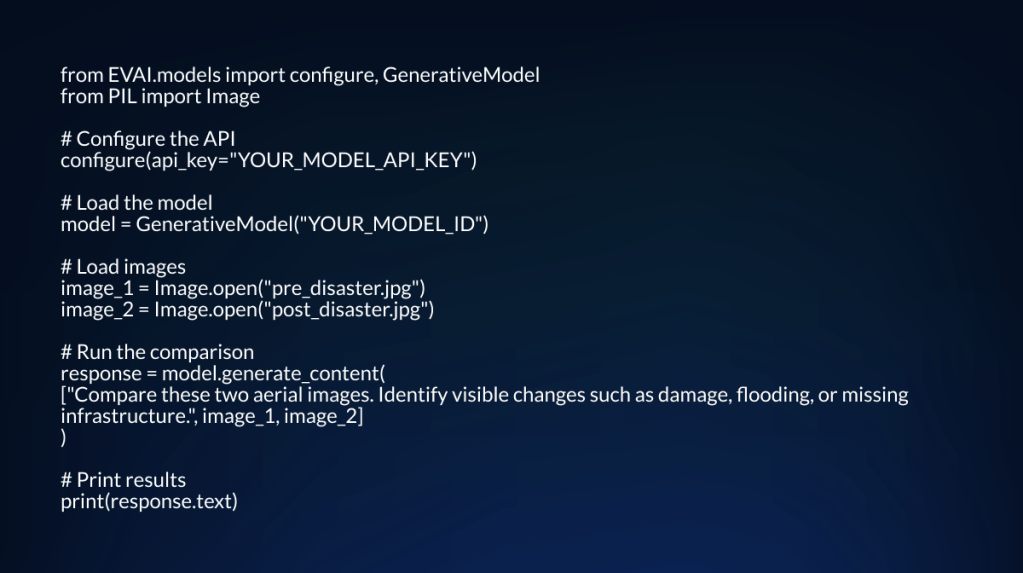
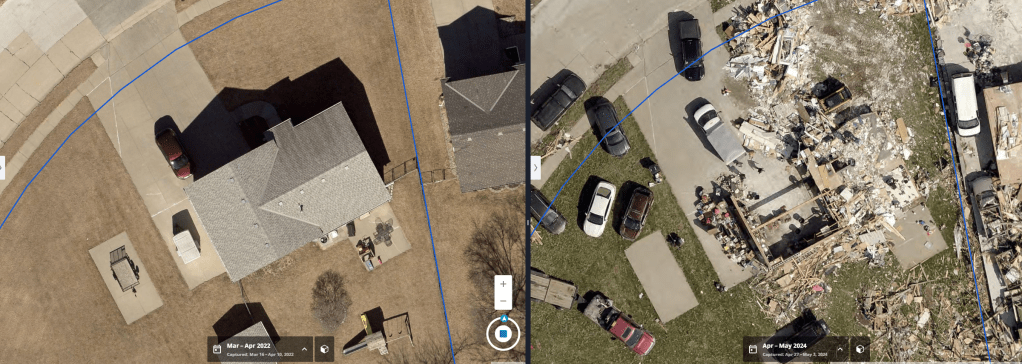
VLM analysis for these images:
Comparing the two aerial images, significant structural damage is evident. In the earlier image, houses, driveways, and backyard features such as patio furniture and playsets are fully intact. In the more recent image, multiple homes have been reduced to rubble, with roofs and walls completely destroyed. Large amounts of debris are scattered across lots, driveways, and streets, and some property boundaries are obscured by wreckage. Several vehicles appear displaced or partially buried, and one structure has been entirely swept from its foundation. There are no visible signs of flooding, but the extent of physical destruction indicates a high-impact event, likely a tornado or severe windstorm.
Applications in Disaster Response
- Flood Monitoring: Identify submerged areas and estimate water levels from aerial images.
- Earthquake Damage Assessment: Detect collapsed buildings and infrastructure damage.
- Wildfire Impact Analysis: Track burned regions and evaluate environmental damage.
- Hurricane and Storm Damage Assessment: Identify affected homes, roads, and power lines.
- Urban Change Detection: Track construction developments and land use changes over time.
Advantages of Using VLMs for Aerial Imagery Analysis
- Faster Decision-Making: Reduces the time required for manual analysis.
- Multimodal Flexibility: Works with both textual and visual inputs.
- Single-Shot: No need to finetune model for different tasks as these are holistic in nature.
- Scalability: Can analyse large-scale aerial datasets efficiently.
- User-Friendly Interaction: Allows non-experts to query data using natural language.
Conclusion
Vision-Language Models (VLMs) have the potential to revolutionize change detection and disaster response in aerial imagery analysis. By enabling automated, accurate, and interactive assessments, VLMs can significantly enhance disaster preparedness and emergency response. As AI technology continues to evolve, integrating VLMs with aerial monitoring systems will be a key step toward a more resilient and data-driven approach to disaster management.
If you are developing a VLM and require access to imagery and data for fine-tuning, contact EagleView to discuss.